On a fateful day in 2003, about a month away from turning 21, Pancho woke up with a terrible headache. His body began stiffening, his speech was slurred, and he could barely stand upright. In the ambulance on the way to the hospital, the paramedics asked him questions, but Pancho – a name he uses to preserve his privacy – couldn’t get any words out. He was admitted to the ER and given a battery of tests. And then everything went blank.
He came to about a week later. “I tried to move and speak, but I couldn’t,” Pancho recalls. “It was terrifying.” He learned he had suffered a stroke, likely a consequence of injuries from a recent car crash. The stroke, his doctors explained, had occurred in his brainstem, severing communication between his brain and the muscles of his body, including those in his face. It dawned on him that he might never move or speak again.
But Pancho is “a man with a lot of faith,” as he puts it. “I thought I would get better somehow, because God is always here with me.” Before his stroke, his life had revolved around working in the vineyards of Sonoma County, California. Afterward, he was confined to hospitals and later to the nursing home where he resides today. “I was at the mercy of anyone in charge of my care,” Pancho recalls. “The worst thing is I couldn’t talk, and even though I tried to nod and signal someone passing by, I couldn’t say what was wrong.”
Eventually, he was given an electric wheelchair that he could operate with residual movements in his arms. He also learned to use residual movements in his head and neck to control a pointer fastened to a baseball cap, with which he painstakingly tapped out words on a virtual keyboard. “It was very slow, but it worked well, and I was able to communicate,” he notes. (He recently upgraded to a typing system that uses a laser pointer mounted on his glasses, which is how he answered questions for this story.)
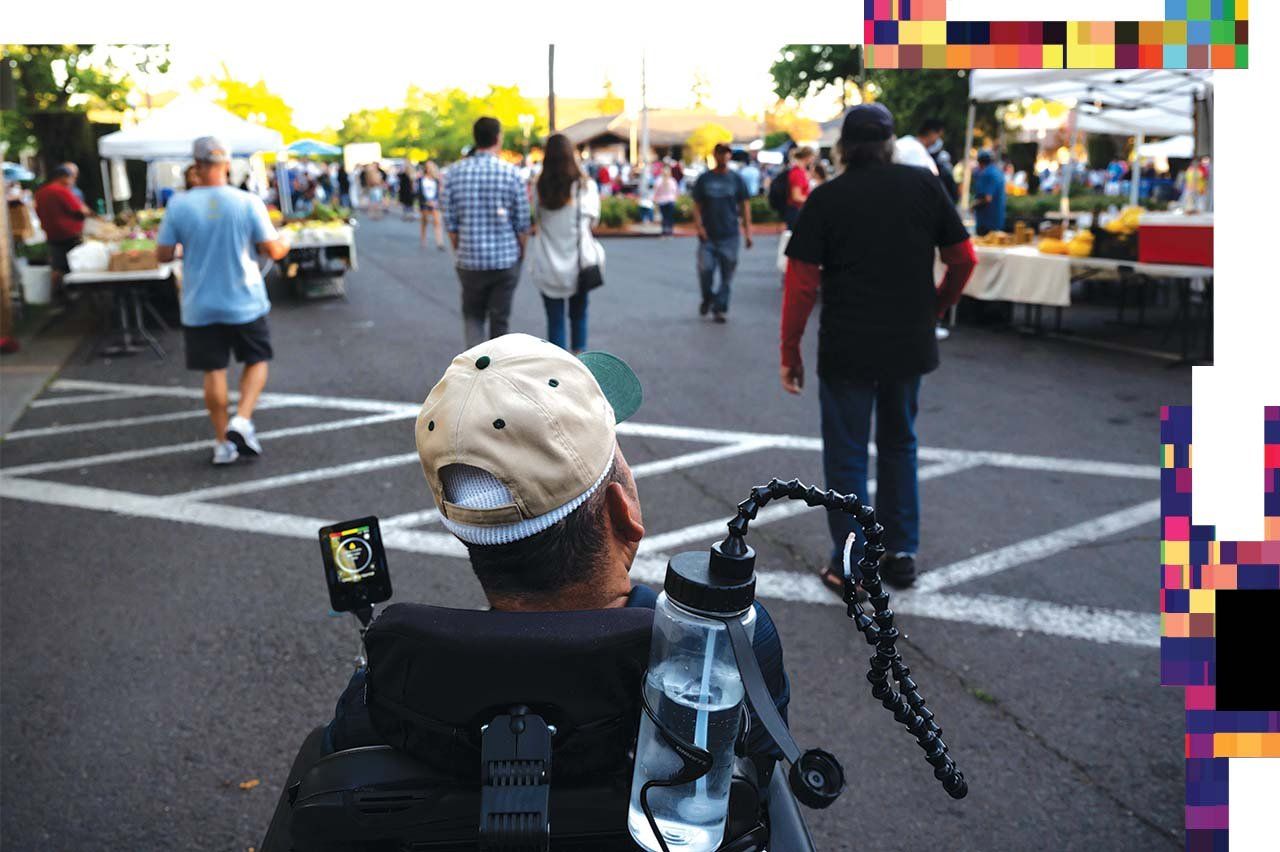
Then, in 2018, Pancho’s neurologist alerted him to an intriguing clinical trial co-led by Edward Chang, MD ’04, a neurosurgeon at UC San Francisco’s Weill Institute for Neurosciences. For about a decade, Chang and his colleagues had been working to understand how the human brain gives rise to speech. They had even learned to transform electrical activity in the brain’s speech center into intelligible sentences. The time had come to attempt the next step: restore speech to someone who had lost the ability to talk. Pancho was the first to volunteer. “I didn’t think twice,” he recalls. (Chang designed the trial with UCSF neurologist Karunesh Ganguly, MD, PhD, whose work focuses on restoring movement in paralyzed patients.)
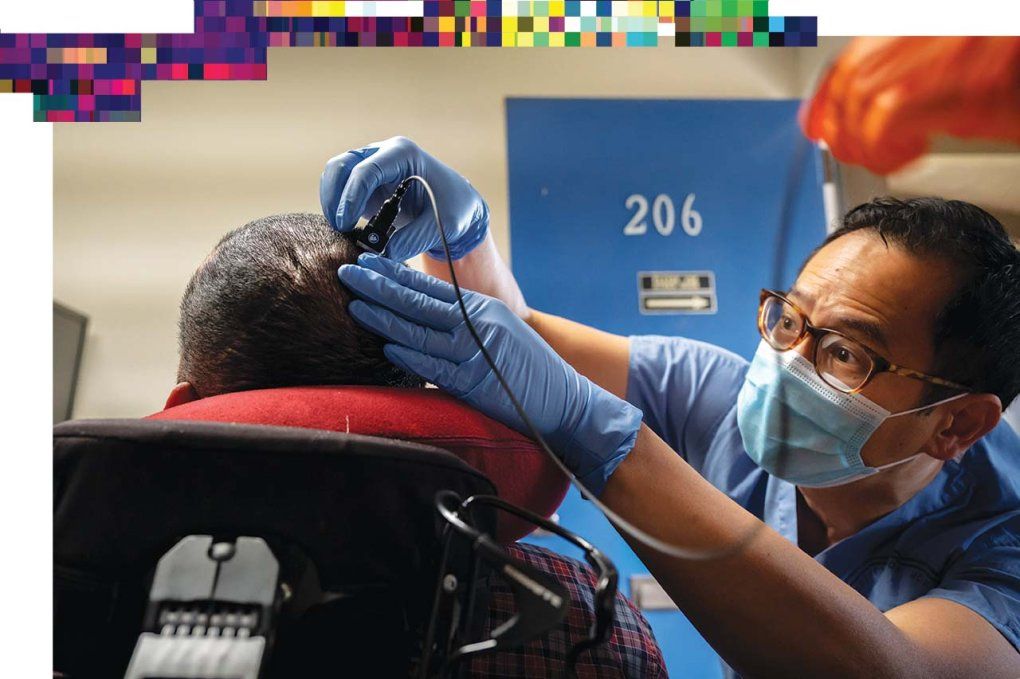
Chang, who is also the chair of Neurological Surgery and the Jeanne Robertson Distinguished Professor of Psychiatry, began the trial by implanting an array of 128 electrodes embedded in a thin silicone sheet beneath Pancho’s skull, on the surface of his brain. These tiny metal discs, which record signals from neurons firing in the brain, connect to a skull-mounted port that breaches Pancho’s scalp, allowing the implant to be plugged into a computer. Chang’s team then set about programming the system, called a neuroprosthesis, to translate Pancho’s brain signals into words.
In July 2021, they announced the results: Pancho became the first person with vocal paralysis to successfully generate words by controlling a speech neuroprosthesis with his brain. Granted, his speech was expressed as text on a computer screen, and he was initially limited to a 50-word vocabulary. But this feat could be the beginning of a sea change for people like him who cannot speak on their own.
The communication tools available to such patients today all rely on typing using eye trackers or other non-brain-based technologies, which are far less efficient than natural speech. The late physicist Stephen Hawking, for example, whose iconic “voice” emanated from a synthesizer that turned text into computer-generated speech, could compose messages at a pace of only a word a minute toward the end of his life. With his laser pointer, Pancho can type about five words a minute. With the neuroprosthesis, however, he can already speak up to 15 words a minute, and, in theory, future generations of the system could enable conversational speeds of about 150 words a minute.
What makes this technology so powerful is that it combines new knowledge about the neuroscience of speech with a form of artificial intelligence called machine learning. Machine-learning algorithms enable researchers to teach computers to recognize complex and sometimes subtle patterns in data that humans are unable to see. These algorithms have proven hugely successful at tasks such as image classification, language translation, and speech recognition (think Siri on your iPhone). Now scientists like those on Chang’s team are using machine learning to augment impaired brains, blurring the boundary between the natural and artificial.
But before Chang’s team could train a machine to help someone like Pancho speak again, they first had to figure out how an unimpaired brain orchestrates the extraordinary array of sounds that make up human speech.
The_Shape_of_Speech
The human vocal tract is a marvel of musculature. When we speak, our brains engage a hundred or so muscles in our jaws, lips, tongues, throats, and lungs. “By themselves, these movements have no meaning,” Chang says, “but when they’re coordinated, like a symphony, they give rise to every consonant and vowel, and from that, every word and sentence. It’s an incredible generative system that allows us to convey an infinite number of messages and meanings.”
Chang’s lab specializes in mapping the patterns of neural activity that control many of the muscles in the vocal tract to produce the sounds of speech. To create these maps, his team has made use of another area of Chang’s expertise: epilepsy surgery. Chang treats patients with drug-resistant epilepsy by excising the brain tissue that causes seizures. To determine what tissue to remove, he temporarily implants an electrode array similar to Pancho’s on the surface of a patient’s brain. He uses the array to both record from and stimulate the brain while the patient is awake, which allows him and his colleagues in the UCSF Epilepsy Center to pinpoint where the seizures originate and steer clear of regions that carry out important functions like language.
Over the past 10 years, more than a hundred epilepsy patients have volunteered to participate in Chang’s speech research. For these studies, researchers record neural signals from the speech center of volunteers’ brains while they speak aloud. In some experiments, the researchers simultaneously monitor the movements of the volunteers’ vocal tract muscles using, for example, video and ultrasound tracking of their lip, tongue, and jaw movements. As a result, Chang’s lab has been able to determine which neural activation patterns correlate to which vocal-tract movements and how these movements form particular sounds.
For example, the team has found that certain neurons fire when the lips close briefly to make the plosive “p” sound in “proof.” Meanwhile, mere millimeters away, different neurons fire when the tongue protrudes forward to make the “th” sound in “that.” Chang’s team has verified such correlations by electrically stimulating these neurons in patients’ brains and observing how the muscles of the vocal tract move in response.
The brain regions involved in speech have been known for over a century. Chang’s lab has not only delineated these regions in more detail but also deciphered the neural code behind every speech sound, or phoneme, in the English language — the DNA of speech, if you will. “It’s a precise spatial-temporal pattern of neural activity that occurs when we speak,” Chang says.
It’s a precise spatial-temporal pattern of neural activity that occurs when we speak.”
In a surprising twist, his team even discovered a second, previously unknown brain region representing the movements of the larynx, or voice box. This region is absent in nonhuman primates, suggesting that it has a unique role in human speech. Indeed, as Chang’s lab later figured out, the newly discovered region helps control the vocal folds in the larynx to change the pitch of a word, which humans often do when we stress a word to change the meaning of a sentence. (For example: “I never said she stole my money” versus “I never said she stole my money.”)
But Chang’s team wasn’t satisfied simply with cracking the neural code of speech movements and sounds. “We’ve always been interested in translating that knowledge to restore function to someone who’s been paralyzed and lost the ability to communicate,” Chang says. He knew, however, that making that leap wouldn’t be easy. For one thing, the precise neural signatures for speech must be reliably identified amid a cacophony of brain signals. For another, speech codes, like genes, vary from person to person. Although they share some common features, no two codes are exactly the same. Accurately transcribing a person’s unique neural signals into their intended phonemes or words, therefore, would require more sophisticated algorithms.
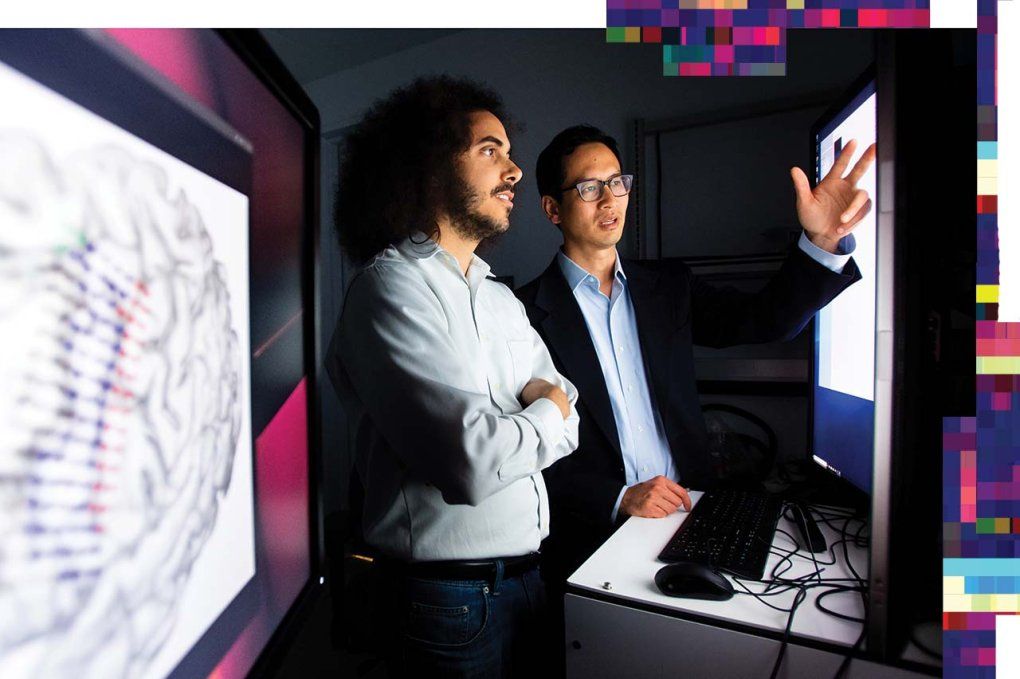
Enter machine learning. Overseeing the development of the algorithms that led to Pancho’s breakthrough was David Moses, PhD ’18. He joined Chang’s lab as a doctoral student in 2013 and then continued on as a postdoctoral fellow. In 2019, Moses and his colleagues published a proof-of-concept study showing that a machine-learning system could be taught to decode phonemes from a person’s brain activity in real time. Three of Chang’s epilepsy patients volunteered for the study. Each volunteer listened to questions and responded aloud with answers that they selected from a multiple-choice list, while the researchers recorded their neural signals. Moses’s team then used these data to train the system using a technique called supervised learning.
In supervised learning, researchers feed into machine-learning algorithms examples of inputs matched with their corresponding outputs. An algorithm tasked with learning to identify cats, for example, might be fed images labeled “cat” or “not cat.” In this way, the algorithm learns to discern the distinguishing features of a cat. In part of Moses’s study, the researchers fed their algorithm each volunteer’s neural signals (inputs) matched with the corresponding audio clips of the volunteer’s speech sounds (outputs). The algorithm thus learned how certain patterns in the signals corresponded to certain phonemes.
Once trained, the machine-learning algorithm could use these correlations to predict the likeliest phoneme a volunteer uttered based solely on their neural activity. Moses wrote another program that then converted those predictions into the likeliest response. His team found that their system could decode a volunteer’s answer to a given question with an accuracy rate of 76% – a significant improvement over chance, at 20%.
Use It_or_Lose It?
Moses’s demonstration and others like it convinced Chang’s team they were on the right track. But the machine-learning systems they had built so far all worked with people whose ability to speak was fully intact. What would happen if they tried to train a system using neural signals from someone like Pancho, who hadn’t spoken for years? Would the brain regions responsible for speech still generate meaningful signals after years of lying fallow?
The brain is plastic: When a region stops being tapped for its original use, the brain can co-opt it for other purposes. By the time Chang’s team began working with Pancho, more than a decade had passed since his stroke. It was entirely possible that the neurons in his brain’s speech center had already been repurposed, erasing their ability to encode the intricate muscle movements that beget intelligible speech.
Even if that weren’t the case, however, Pancho’s disability presented another challenge. “When he tries to say a word, it comes out like a grunt,” Moses says, or like a moan. “It’s much harder to tell exactly when each phoneme occurs,” he adds, than it is with speaking volunteers. Training a machine-learning system to identify phonemes from Pancho’s neural signals would therefore be difficult. So instead, the team – which by then included graduate students Jessie Liu and Sean Metzger – decided to try decoding entire words, since it’s easier to tell when Pancho is trying to say a word than a phoneme.
For that, they turned to the most powerful form of machine learning: deep neural networks. Inspired by neural networks in the brain, deep neural networks, or deep nets for short, consist of layers of artificial neurons – simplified computational models of biological neurons. When a deep net is fed training data (pairs of inputs and outputs), it strengthens or weakens the connections between these copycat neurons, analogous to how the human brain tunes the synapses between biological neurons when we learn.
Typically, deep nets are data-hungry hogs: Give them enough training examples, and they’ll learn patterns in just about anything. They’re superb at tasks like identifying images or voice commands, mainly because internet companies have amassed decades of image and voice data, as well as the massive computing resources needed to store and process them. But Chang’s team didn’t have those luxuries. “When you are working with someone who is paralyzed, you’re very data constrained,” Chang says.
What the team did have, however, was more than a decade’s worth of knowledge about the neural basis of speech. They could thus use that knowledge to enable a machine-learning system to decode Pancho’s brain signals with fewer training data by first using filters to extract content in the relevant frequency range. The team then used the filtered signals to train a deep net to recognize when Pancho was attempting to speak and identify brief time segments of neural activity representing words.
Next, the researchers fed those time segments into another deep net, which learned to decipher the likeliest word being spoken. With Pancho’s help, the team taught this algorithm to recognize 50 common words from his brain signals, including “hello” and “thirsty.” For each time segment of neural activity, the algorithm spit out 50 numbers signifying the probabilities that Pancho was trying to say each of the 50 words.
Finally, the team trained a third machine-learning algorithm called a language model to convert a series of word probabilities into the most likely sentence. For example, if the second deep net predicts that a word Pancho is attempting to say is equally likely to be “hello” or “thirsty,” but his previous words were most likely “I” and “am,” then the language model would output the sentence “I am thirsty.”
After months of training, the AI powering Pancho’s neuroprosthesis was ready. All that remained was for Pancho to test it out.
A_Voice_of_His Own
“My family is outside.”
Pancho remembers that this was the first sentence he spoke through his neuroprosthesis. The words appeared almost immediately on the computer screen before him. “I was so excited, I couldn’t believe it,” he recalls. “Whenever I get excited about something, I have a spontaneous laughter – a horrible laugh that comes out of nowhere and messes everything up.” Nevertheless, he adds, “I was able to pull myself together and keep going.”
I was so excited, I couldn’t believe it.”
Since completing the 50-word study, Chang and his team have continued to work with Pancho, visiting him at his nursing home in Sonoma two days a week. They are experimenting with ways to make the neuroprosthesis faster and more accurate. In the study, for instance, the team reported an average error rate of 26%. They have since found that they can reduce that rate if Pancho attempts to speak without grunting. Simply speaking through his mind, and stopping short of vocalizing, is both easier for him and more effective. “In his mind, he’s more free,” Chang says, echoing something Hawking wrote about his own travails: “Although I cannot move and I have to speak through a computer, in my mind I am free.”
The team is also working to increase Pancho’s vocabulary. But expanding to even 100 words isn’t trivial. “You have to collect tons and tons more data each time a new word is added,” Moses explains. With more than 150,000 words in the English language, it’s unlikely that a neuroprosthesis based on decoding words directly could ever give someone like Pancho back a full English vocabulary, let alone a bilingual one. (Pancho is a native Spanish speaker.)
A more scalable approach would be to build a phoneme-based system like the one Moses built in his 2019 study. That’s a much tougher bioengineering task given Pancho’s inability to clearly articulate sounds. But because English has only 44 distinct phonemes, a neuroprosthesis trained to decode all 44 would be able to piece together any word a person wants to say. “You could theoretically decode words that a person is trying to say even if they never actually said those words before,” Moses says – including newly minted words.
Meanwhile, Chang’s team is exploring a third approach that involves decoding intended movements of the vocal tract to create synthesized speech rather than text. “One of our bigger goals is to actually have audible words come out,” Chang says. A recent study led by Gopala Anumanchipalli, PhD, an assistant professor, and Josh Chartier, PhD, a postdoc in Chang’s lab, demonstrated such a system with epileptic volunteers. Remarkably, the system could synthesize speech from the volunteers’ neural signals even when they silently mouthed the words.
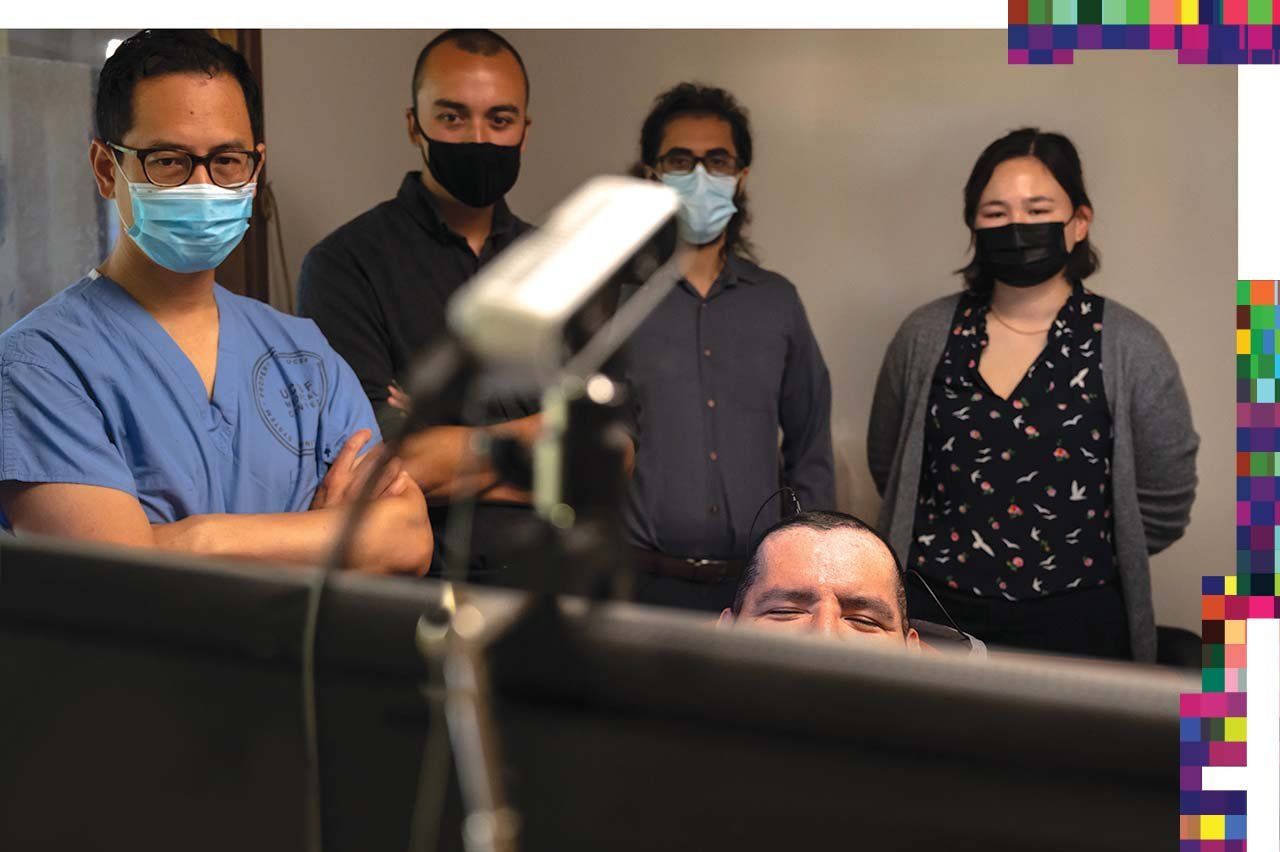
Chang envisages still more futuristic enhancements. With advances in hardware, he believes, implantable electrode arrays will soon be able to communicate with computers wirelessly. He imagines restoring speech not just in one language but two or more, for multilingual patients. He thinks about children with cerebral palsy, who have never been able to speak, and wonders if a neuroprosthesis similar to Pancho’s could help them, too. He speculates about implanting a second set of electrodes in a paralyzed person’s vocal tract muscles to stimulate them using commands from electrodes in their brain, letting them speak again in their own voice.
Inevitably, people always ask Chang if his lab’s technology will one day be able to read private thoughts. “In a theoretical sense, if the brain is truly the source of our thoughts, concepts, and knowledge, then it’s absolutely possible,” Chang says. Pancho’s neuroprosthesis, however, doesn’t even come close, he points out. “He can’t just be thinking about a word or imagining it. It’s got to be a volitional act.” Still, Chang concedes, “it’s worth talking through the potential implications now.”
For his part, Pancho can’t wait for the day when he can use his speech neuroprosthesis in everyday life. “I am very hopeful about that,” he says. “It is going to be something so amazing, I can’t even describe it. It blows my mind just to think about it.”