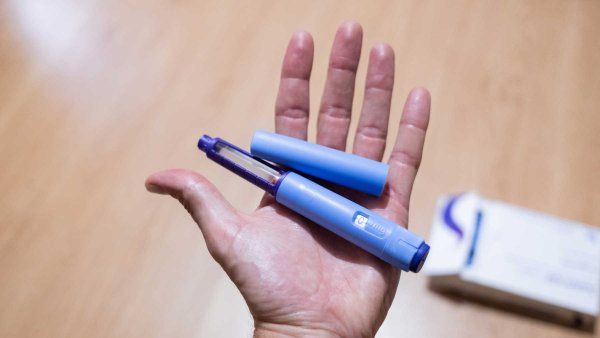
A better cure for cancer – and other illnesses – could already be in existence, hidden right under our noses.
The problem is that possible new lifesaving drugs are created much faster than scientists can study them. Millions of untested compounds wait, jumbled together in no particular order in vast repositories called compound libraries.
“These libraries are basically like black boxes right now,” says Steven Altschuler, PhD, a professor of pharmaceutical chemistry at UC San Francisco’s School of Pharmacy and member of the UCSF Helen Diller Family Comprehensive Cancer Center.
“You imagine that somewhere in there is some chemical that might be the key to unlocking any question that you have – but how are you going to find it?”
A new search method that blends cellular biology and computational analytics may be the answer. A husband-and-wife research team at UCSF – Altschuler and his longtime spouse and collaborator, Lani Wu, PhD, also a professor of pharmaceutical chemistry and member of the cancer center – have developed a way to do the job much faster and at a fraction of the cost of the traditional method. The work involved designing a new kind of cell, writing some new software, and then parsing the resulting landslide of data.
“We were very lucky to be there at the right time and see the connections,” says Altschuler. “Going in, we didn’t even realize that there was a need for this.”
The couple was uniquely poised to develop this method, as their work is informed not only by their current collaboration but also by their earlier shared careers in other fields. They have worked together since they met as students almost 30 years ago.
“We met in the mailroom,” Altschuler says. “It was the first day of grad school for her; I was a second-year.”
The pair started out their parallel careers in mathematics and went on to work for Microsoft, then for a biotech firm, before moving into academia.
“Most of us wouldn’t even think of an analogy between drug discovery and what they were working on at Microsoft with image recognition and things like that,” says Matthew Jacobson, PhD, chair of the Department of Pharmaceutical Chemistry, who recruited Altschuler and Wu to UCSF. “To me, this just shows the power of bringing people with different types of backgrounds into biology and drug discovery.”

Too Much of A Good Thing
Screening compounds for potential medical uses has to date been both time-consuming and expensive. For example, a lab looking to develop better chemotherapeutic agents would likely be interested in DNA-damaging drugs that have yet to be tested. Usually, researchers are looking for drugs that affect the chain of cellular events by which a given disease advances or can be treated – a biochemical process known as a pathway. An unknown number of such compounds are likely available in libraries housed at universities and pharmaceutical companies around the country. But how to find them?
“Over the last few decades, drug discovery has tended to be fairly pragmatic,” says Jacobson. “We tend to make various simplifying assumptions about how things work inside cells.”
Often, scientists search for new drugs using carefully engineered “reporter cells” to screen for sought-after compounds. These cells are designed in a lab to change in a unique way when a compound achieves a desired effect, indicating to researchers that they have a match. However, this method tests for just one purpose at a time – and it costs hundreds of dollars to test each “mystery” compound. This means researchers generally can afford to screen only a small, random subset of the available compounds.
Envision a compound library as a massive box containing thousands of unorganized, unlabeled photographs. The current method is akin to each researcher pulling out a handful of photos and looking through each handful to find pictures of one particular person. They may be able to identify a few, but every future project has to start from scratch.
“Whenever anyone goes in there with a specific question, they might find 12 chemicals that are interesting to them, and the rest just go right back into the bin,” Altschuler says.

A New Way Forward
By contrast, imagine a method that digitizes the photos and then screens them with a program akin to facial recognition software. This is the first step in Altschuler and Wu’s new method, which categorizes reporter cells in much the same way that Facebook tags photos of your friends: by digitally identifying their features.
Now, when a compound library with unknown properties is screened with reporter cells, the software can identify which of those compounds is generating a desired response. The cost for each test drops from hundreds of dollars to a dollar or less, Altschuler says.
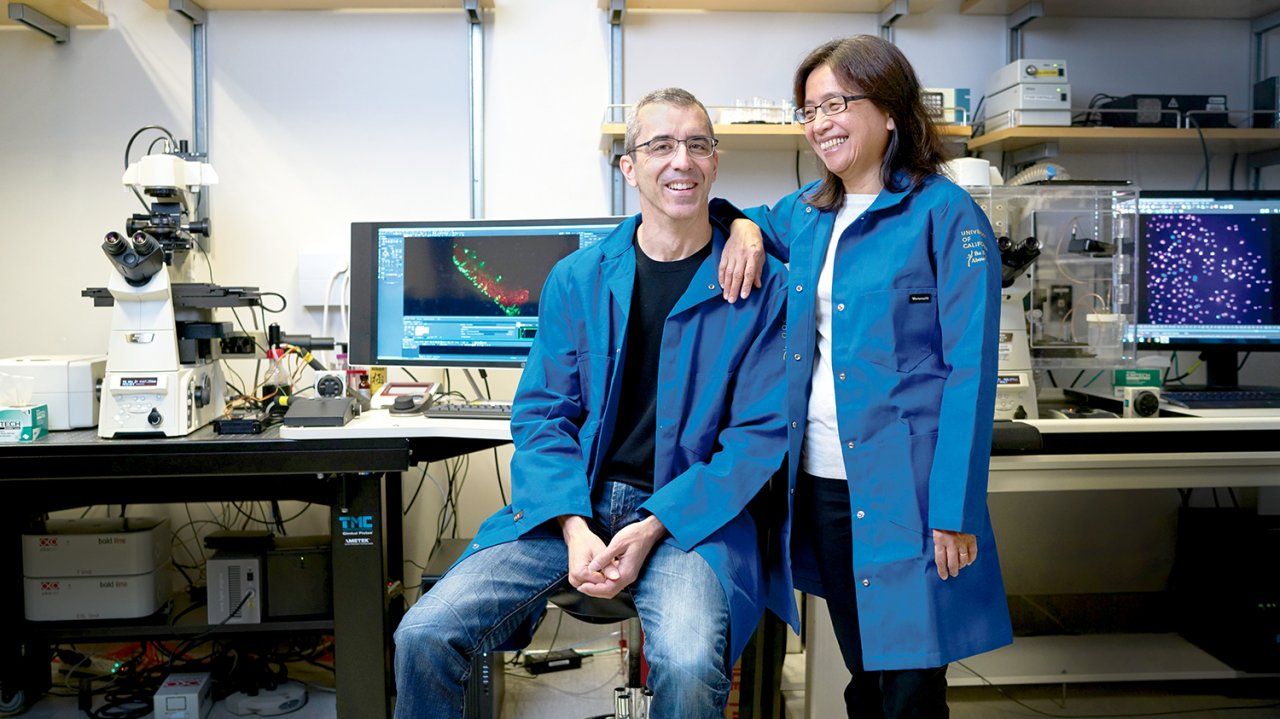
Husband-and-wife research team Steven Altschuler and Lani Wu started out their careers in mathematics. Photo: Steve Babuljak
That was phase one of the work. More recently, the couple has found a way to test drugs for multiple purposes at a time using this same principle. An experiment that their team described in Nature Biotechnology this past January used only one type of reporter cell to screen nearly 11,000 drugs from multiple compound libraries for six disease pathways. That’s like using facial recognition software to digitally sort through hundreds of thousands of photos – think of multiple boxes stored in Grandma’s attic – and tag photos of six different people at once. Up until now, researchers would have had to do one experiment just to find photos of Steve, a separate one to find photos of Lani, and so on.
“The ability to do very sophisticated mathematical analyses basically allows them to identify the effects of drugs much more sensitively than other approaches have been able to do,” Jacobson says.
This may sound straightforward enough, but consider the fact that researchers had previously found it difficult to coax a single reporter cell into partitioning multiple unidentified drugs into categories of usefulness. Yet Altschuler and Wu not only did that but also yielded results that can be processed digitally – making it clear that this is a quiet but revolutionary breakthrough.
“The grand challenge here is really trying to understand how administering a drug to a cell affects not just one individual protein but the entire network of proteins and, ultimately, the cell’s behavior,” Jacobson says.

Seeking an ORACL
Standard reporter cells essentially work like matching cards in the children’s game known as Memory: when a new compound generates a response that resembles that of a known drug, it tells researchers that the two operate on a similar pathway and are likely to affect the target disease in a similar way. Over the last decade, such genetically encoded reporter cells have become increasingly popular in biotech research.
But Altschuler and Wu wanted to design a single, versatile type of engineered cell that would report when a compound matched multiple different pathways – something that had never been done before. Instead of trying to reason their way to a solution, the team decided to seek a boost from chance: Using the fluorescent tags that are used to build reporter cells, they put roughly 600 cells through the DNA tagging process – but did not attempt to control where the tags landed.
They hit the jackpot. In their initial pool of potential reporter cells, they found one that was 94-percent accurate in discriminating among six diverse cancer-relevant drug classes. They named it ORACL, which stands for Optimal Reporter cell lines for Annotating Compound Libraries.
“They just randomly tried stuff and found that there is a tremendous amount of information in a very small number of proteins – you don’t even have to really think too hard about what they are,” Jacobson says.
The challenge with such a versatile reporter is to know what it’s saying. The human eye can distinguish only some of a reporter cell’s responses – for example, the way DNA-damaging drugs make the reporter swell up or the way certain cellular inhibitors make it grow long, spiky arms. But other changes can be identified only by computer – which is also the only way to parse the sheer volume of data.
This is a trend that has been on the rise throughout the field. “Data science is a big part of biology right now,” Wu says.
“No human being could look at this,” Altschuler says. “The changes are too subtle, the numbers of conditions are too large, for any human to assess. This really required innovations in identifying cells, extracting properties of the cells, and comparing how the cells had changed in different conditions.”
A senior researcher in the lab tested the process before the machines took over. Jungseog Kang, PhD, dripped nearly 11,000 compounds and 38 reference drugs into the waiting mouths of tiny wells full of ORACL cells. Once the cells had reacted to the compounds, they were photographed through a microscope. Then, photos were analyzed by software developed by a graduate student, Charles Hsu, producing digital profiles that were matched to those of reference drugs. The results were cross-checked by a literature review and experimentation. In the end, the method proved 94 percent accurate.
“A lot of the magic is in the software, [in] being able to extract maximal information out of a minimal number of experiments,” Jacobson says. “Basically, they are asking, ‘Might it do something interesting and useful? And if so, does is it look like anything we’ve seen before? Or is it something totally new?’ And both categories are interesting.”
The ORACL found 100 new compounds that fit one of six drug classes. And it had still more to reveal. To the team’s surprise, additional clusters of potentially useful compounds were identified, despite not being in the experiment design – including glucocorticoids and ATPase inhibitors, which can treat autoimmune conditions and cancer, respectively.
“That was really cool,” Altschuler says. “That means it’s a way to go fishing even beyond what we thought we were going to catch.”

Tip of the Iceberg
Seated at a sterile stainless steel hood, postdoctoral scholar Louise Evans, PhD, has taken over from Kang. Sliding a bristling phalanx of nearly 100 pipettes back and forth on a mechanical arm, she is helping move the ORACL on to its next step. Compared to the number of compounds that remain uncategorized, this experiment’s 10,000-compound sample is the tip of the iceberg.
“Once you get into the hundred-thousand- to a million-compound range, that would be considered pretty interesting for a phenotypic screen in academics,” Altschuler says. “But I’m not sure that the size of the library you screen is the most important thing.”
It’s actually not that different from maybe a music recommender. Music gets classified, and you say what genre you like, and it tries to bring back some more like that.”
The vision is that in the future, the ORACL method will lead to compound libraries that are indexed and searchable according to each compound’s effect – instead of being the black boxes that they are today.
“In principle, we hope a researcher can come to us with a type of compound they are interested in, and we can go into our database and say, ‘We’re going to recommend a few different ones that are just like it for you,’” Altschuler says. “It’s actually not that different from maybe a music recommender. Music gets classified, and you say what genre you like, and it tries to bring back some more like that.”
The next step is likely a partnership with the private sector, where there are the resources to test the method on a larger scale.
“There is a hunger in the pharma companies for new approaches, a willingness to try things,” says Jacobson. “Drug discovery is a long and exceedingly painful process. In the short run, the impacts will come through just scaling up this new approach. I think in the next few years, it will come clear whether this is going to be widely adopted or not.”