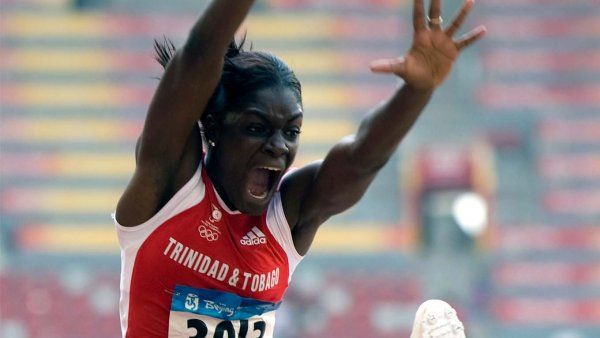
Big Data: Big Deal
Q&A with data scientist Atul Butte
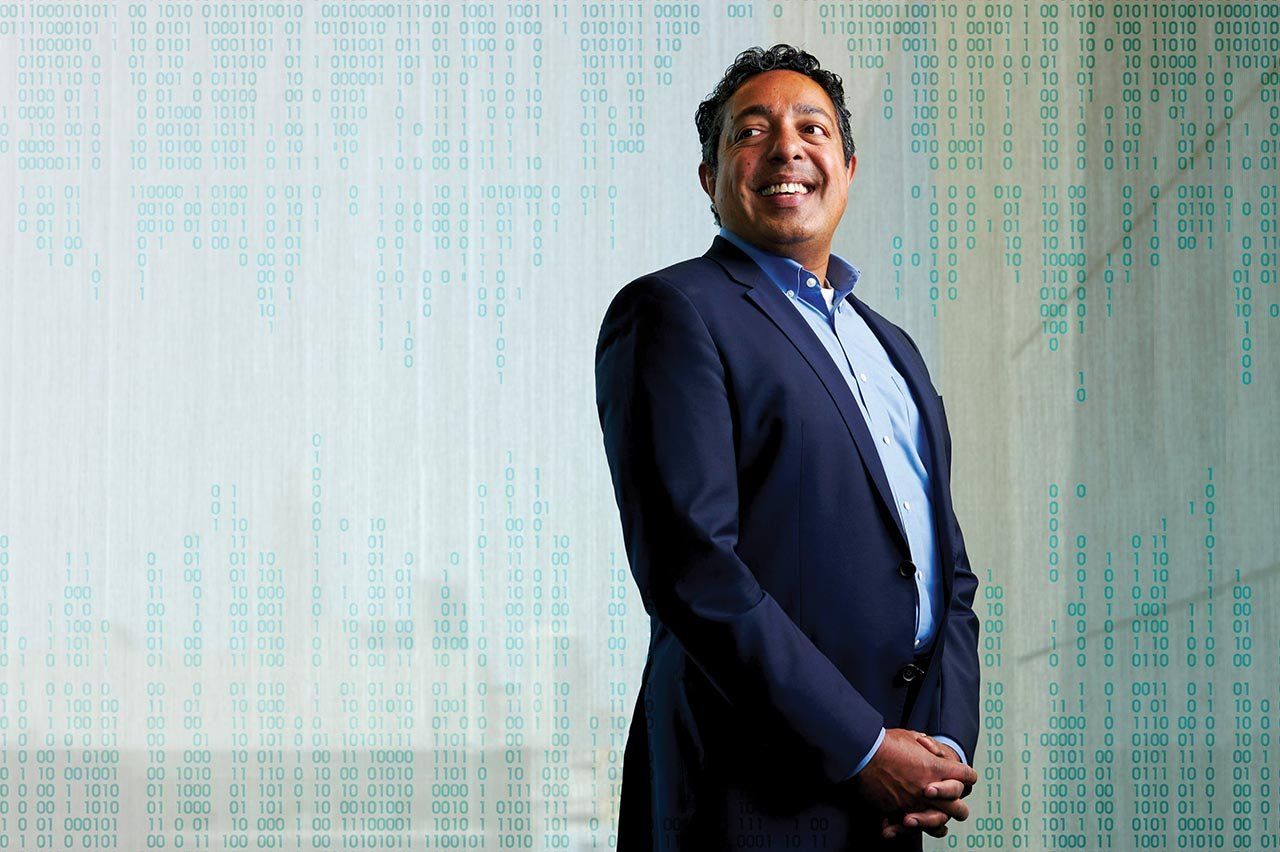
Photo: Steve Babuljak
Atul Butte, MD, PhD, wears many hats at UCSF: professor of pediatrics, director of the UCSF Bakar Computational Health Sciences Institute, and the Priscilla Chan and Mark Zuckerberg Distinguished Professor. He is also the chief data scientist for UC Health, the infrastructure uniting all six UC medical centers: UCSF, UC Davis, UC Irvine, UCLA, UC Riverside, and UC San Diego. Butte and his team are harnessing the collective power of UC’s systemwide biomedical data – which he sees as a first step in building a massive global database that will someday enable precise, targeted, accountable care in California and around the world.
What kind of data are we talking about?
Some data is easy to get at – like electronic medical records [EMRs], lab results, admissions notes. Some is more scattered – like DNA samples, gene expression data, clinical trial records. We’ve invested millions of dollars in EMR systems and cool gizmos like wearable medical devices to generate more and more data. Much of it is accessible with the right governance and permissions, and it’s more than enough to make a difference. But we’re really not doing much with it at this point. Data by itself does nothing. We have to turn it into knowledge to effect changes in policy and behavior. All this data is just sitting there, waiting for us to ask the right questions.
How do we make sense of it?
We need to train people to ask, “What can I do with it?” Biomedical big data is, by definition, big, raw, and messy. The more we have, the more amazing it is. But the hard part is figuring out what to do with it. The solution is to educate – and inspire – more data scientists, people trained in biomedical and computer sciences and statistics. Companies offer high salaries to snap up these folks, so it takes dedication for them to stay in academia. We might need to start training and recruiting even earlier, in high school.
Data drives discovery at UCSF
Here are three of the projects that researchers at the Institute for Computational Health Sciences are working on:
Passion can make a curious researcher even more curious. Bin Chen, PhD, is a native of China, where there’s a high incidence of hepatocellular carcinoma (HCC), a leading cause of cancer deaths. Searching through publicly available gene expression data, he found 274 genes that regulate cancerous liver tissue. Then, among drugs known to target those genes, he discovered a deworming pill that, in combination with standard HCC therapeutics, was highly effective at killing cancerous tissue. The approach could help turn HCC from a lethal disease into a chronic condition.
Could the ubiquitous smartphone serve as a tool for managing health? Absolutely, says Ida Sim, MD, PhD, who studies mobile apps and sensors designed to track patient data. Users get real-time feedback to help them manage chronic conditions and maintain better health; they can also share the data with their physicians. Sim cofounded Open mHealth, a nonprofit that supports mobile app and data integration through an open software architecture. She is also developing data-sharing methods that could speed up clinical trials and bring down their cost.
Each year, 15 million babies worldwide are born prematurely, putting them at risk for serious, lifelong complications. Marina Sirota, PhD, is part of a multidisciplinary team that’s investigating immunity – specifically, the tolerance mechanisms that prevent maternal and fetal immune systems from rejecting each other in healthy pregnancies. Sirota’s lab is also building an integrated data repository for collaborative studies on adverse pregnancy outcomes and advancing management and prevention strategies by studying genetic, environmental, and clinical factors that may contribute to preterm birth.
What kinds of problems can you solve with big data?
Say you’re researching a treatment for liver cancer. You could start with millions of chemicals and petri dishes full of cells and eventually get a drug into a clinical trial, which costs a billion dollars and takes 15 years. But start with the data instead, and a dedicated researcher can launch a data-driven experiment for just $50,000. In fact, ICHS researcher Bin Chen did exactly that for hepatocellular carcinoma. It’s known as drug repositioning: We take data on tens of thousands of drugs – some already approved for human use – and match them with gene expression data on a given disease, looking for drugs made for another purpose that can affect this disease. It’s like Match.com for drugs. Data can also lead us to other solutions, like designing a more specific blood test, or eliminating unnecessary blood transfusions, or creating maps of disease and death that show us how a disease will behave over time.
Where is all this leading us?
If we want to change the world, we need to do something with our data and discoveries; we can’t just keep writing papers. For example, the ICHS is working with all six UC medical centers to aggregate 15 million patient records – strictly regulated to safeguard privacy – into one safe, secure, reliable repository. There’s no other set of academic medical systems in the U.S. with as much patient data and as much computing power to analyze it as UC has. This is where we need to start if we are going to get all our data in one place. Not just UCSF’s data, not just UC’s data, but everyone’s data – what care we provided, what worked, what didn’t work. We can then predict what will happen with any given patient or any given disease in any environment over the next 90 days or year or 10 years. We’ve got to get there so we can provide truly customized, precise, and accountable medical care for everyone.